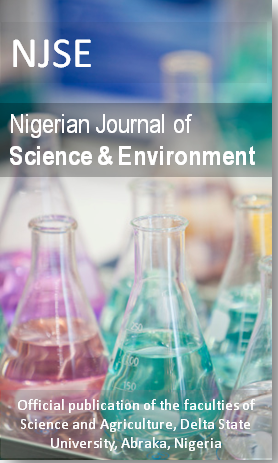
NIGERIAN JOURNAL OF SCIENCE AND ENVIRONMENT
Journal of the Faculties of Science and Agriculture, Delta State University, Abraka, Nigeria
ISSN: 1119-9008
DOI: 10.5987/UJ-NJSE
Email: njse@universityjournals.org
BOOSTING AND BAGGING IN KERNEL DENSITY ESTIMATION
DOI: 10.5987/UJ-NJSE.16.055.1 | Article Number: 3F6EC26 | Vol.14 (1) - July 2016
Authors: , Siloko I. U. and Ishiekwene C. C.
Keywords: Smoothing parameter, bias, variance, boosting, bagging, asymptotic mean integration squared error (AMISE), weak learners
Boosting is a bias reduction technique while bagging is a variance reduction method. These two methods aim at reducing the asymptotic mean integrated square error (AMISE). This study aims to show that bagging is a boosting algorithm in kernel density estimation since both techniques use large smoothing parameter(s). This relationship was verified by real and simulated data
Breiman, L. (2001). Using Iterated Bagging to Debias Regressions.Machine Learning. 45(3):261–277.
Breiman, L. (1998). Arcing Classifiers. AS 26(3):801–849.
Breiman, L. (1996). Bagging Predictors. Machine Learning.26:123–140.
Buhlmann, P. and Yu, B. (2003). Boosting With the L2 Loss: Regression and Classification. Journal of the American Statistical Association. 98:324–339.
Freund, Y. and Schapire, R. (1995).A Decision-Theoretic Generalization of On-Line Learning and an Application to Boosting.European Conference on Computational Learning Theory. pp. 23–37.
Friedman, J., Hastie, T. and Tibshirani, R. (2000). Additive Logistics Regression: A statistical View of Boosting (With Discussion). Annals of Statistics. 28(2):337–374.
Gey, S. and Poggi, J. M. (2006). Boosting and Instability for Regression Trees. Computational Statistics and Data Analysis. 50:533–550.
Ishiekwene, C. C. (2008). Bias Reduction Techniques in KDE. An Unpublished Ph.D Thesis Submitted to the School of Postgraduate Studies, University of Benin, Benin City, Nigeria.
Marzio, D.M. and Taylor, C.C (2004).“Boosting Kernel Density Estimates: A Bias Reduction Technique? ˮ Biometrika 91:226–233.
Marzio, D.M. and Taylor, C.C (2005). "On Boosting Kernel Density Methods for Multivariate Data: Density Estimation and Classificationˮ, Statistical Methods and Applications. 14:163–178.
Mason, L., Baxter, J., Bartlett, P. and Frean, M. (1999). Boosting Algorithms as Gradient Descent.Neural Information Processing Systems, Vol. 12.
Ridgeway, G. (2002). Looking for Lumps: Boosting and Bagging for Density Estimation. Comput.Stat. Data Anal.38(4):379–392.
Rosset, S. (2003).Topics in Regularization and Boosting. A Dissertation Submitted to the Department of Statistics and the Committee on Graduate Studies of Stanford University.
Rosset, S., Zhu, J. and Hastie, T. (2004). Boosting as a Regularized Path to a Maximum Margin Classifier. Journal of Machine Learning Research. 5:941–973.
Sain, R.S. (2002). Multivariate Locally Adaptive Density Estimation. Computational Statistics and Data Analysis. 39:165–186.
Scott, D.W. (1992). Multivariate Density Estimation. Theory, Practice and Visualisation. Wiley, New York.
Silverman, B.W. (1986). Density Estimation for Statistics and Data Analysis. Chapman and Hall, London.