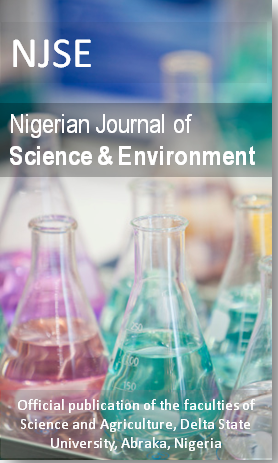
NIGERIAN JOURNAL OF SCIENCE AND ENVIRONMENT
Journal of the Faculties of Science and Agriculture, Delta State University, Abraka, Nigeria
ISSN: 1119-9008
DOI: 10.5987/UJ-NJSE
Email: njse@universityjournals.org
SUPERVISE DIFFERENCE LINEAR CLASSIFICATION TECHNIQUE FOR TWO GROUP’S PROBLEM
DOI: 10.5987/UJ-NJSE.16.067.1 | Article Number: A35A3918 | Vol.14 (1) - July 2016
Author: Okwonu F. Z.
Keywords: Mean probability, sample size, hypothesized mean, robust.
Classification techniques can be categorized as parametric and nonparametric. The former strictly depends on assumptions, and is a supervised linear classification procedure whereas the later does not rely on any assumptions and is an unsupervised linear classification technique. This paper considers supervised linear classification techniques in which the course of dimensionality is negated. In this regard, the sample size is greater than the sample dimension. We investigated the performance of the Fisher, and the difference linear classification techniques based on the classification performance and the acceptance or rejection of the null or alternative hypothesis in which the mean of the optimal probability of correct classification is used as the hypothesized mean, and the computed mean probability is derived from the mean probability of correct classification based on 1000 replications. The robustness of these techniques is determined by the sensitivity of these methods to contaminated data set. Relying on the performance analysis, we further investigated the acceptance or rejection of the null or alternate hypothesis based on the proportion of contamination using the Hoteling test statistics. The comparative analysis indicates that the difference linear classification rule outperformed the conventional Fisher’s technique. The analysis revealed that using 95% level of significant, the null hypothesis is rejected.
Bouveyron, C., (2013). Probabilistic model-based discriminant analysis and clustering methods in chemometrics. Journal of Chemometrics In press, Pp. 1-21.
Fisher, R. A., (1936). The use of multiple measurements in taxonomic problems. Annals of Eugenics, Pp.179 - 188.
Okwonu, F. Z. and Othman, A. R., (2013). Heteroscedastic variance covariance matrices for unbiased two groups linear classification methods. Applied Mathematical Sciences, Pp.6855-6865.
Okwonu, F. Z. and Othman, A. R. (2014). Effect of heteroscedastic variance covariance matrices on two groups linear classification techniques. Journal of Mathematics and System Science, Pp.133-138.
Rencher, A. C., (2002). A methods of multivariate analysis. A John Wiley & Sons, Inc.
Johnson, R. A. and Wichern, D. W., (2007). Applied multivariate statistical analysis. Pearson Prentice Hall, Upper Saddle River.